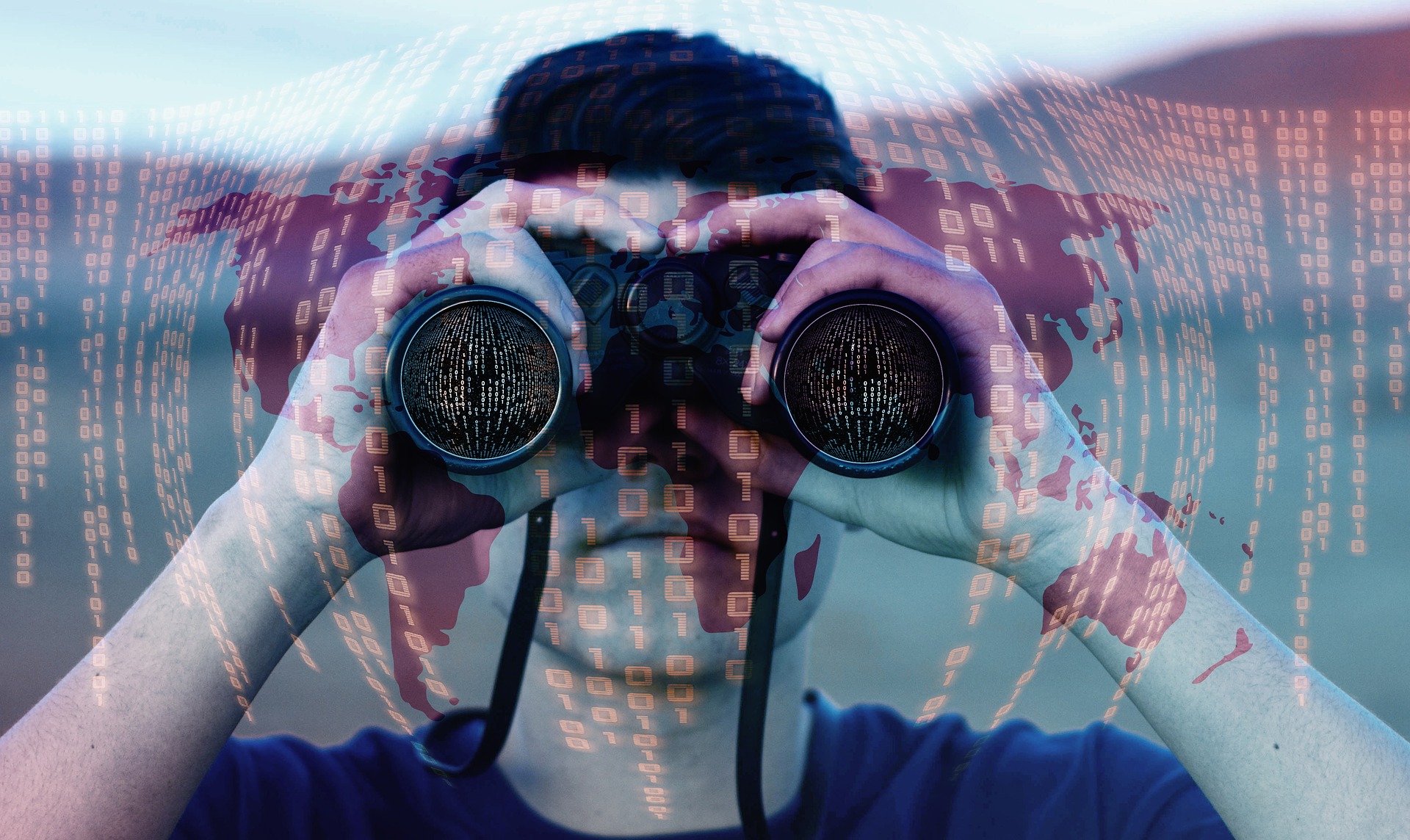
Forecasting – How low should you go?
Companies need to be able to forecast demand accurately to avoid unnecessary losses. But strangely, there is no hard-and-fast method for doing this. It all depends on the level at which you treat the data.
Going High And Low
Traditionally, companies broke down forecasting into two different paradigms: bottom-up or top-down.
The bottom-up approach starts at the SKU level and then builds a forecast around that. Demand planners consult with each other and build statistical models based on prior line data to predict how demand might change over the coming month. It’s a high-effort process, but firms hope the increased precision is worth it.
Top-down forecasting is different and requires less work. Here, central planners aggregate all the data at a high level and then develop a summary forecast of how they expect demand to pan out over the following month.
The top-down approach increases the signal-to-noise ratio at the expense of granularity. When you aggregate forecasts together, you wash out some of the item-by-item variations by the law of large numbers. However, you get less insight into what’s happening lower down the stack.
When lower-level data is relatively homogenous and highly correlated, top-down aggregations are relatively accurate. The headline figure you get is broadly representative of what’s going on at the base level. But when the data are independent and highly varied, then that stops being the case. The headline figure masks the heterogeneity lurking beneath.
You might think that the answer would be to perform bottom-up planning every time, but that’s not the case. Planning at the SKU level is highly volatile, meaning that you won’t always get statistically robust forecasts, even if you take into account all the available data. Going too granular may lead to a paucity of data points, leading to less precise forecasts and missed signals.
There are other problems too. You might have a set of plausible forecasts at the lower level, but once you aggregate them, you wind up with a demand plan that is at odds with realities, due to inaccuracies lower down. Essentially, you’re building a house on the sand.
So how low should you go?
Necessary Detail Versus Unnecessary Complexity
The solution is to attack the problem in a new way.
We suggest running forecasts at different levels and then prorating them down through the various layers of abstraction to see whether there is broad agreement between the figures.
Pick some attributes at random places in the hierarchy and then work through the models to see whether there is a rough agreement between the figures. If the base-level data are relatively homogenous, you should end up with results that roughly agree with each other. If not, then coming at the issue from different angles can tell you if there is a discrepancy.
In an optimal demand forecasting approach, you balance necessarily detail without allowing your modelling to become overly complex. When “going lower,” ask yourself if the added detail is allowing you to get closer to your overall objectives. Be honest with yourself about whether you have the resources available to conduct such a sophisticated project. And be clear that the added detail won’t become a distraction that gets in the way of your goals.
Choosing Multiple Attributes Up And Down The Hierarchy
Going lowers requires being honest about the quality of your data. While high-level analysis might behave predictably, you may not have good data points at the item-level to create bottom-up forecasts that make sense. Demand for some SKUs can be sparse or unpredictable.
Your best bet is to take the middle line. Choose an attribute halfway up the hierarchy – say the customer or area – and then use that to work out what is likely to happen both above and below. Once you have done that, check to see whether it corresponds to your expected headline figure for the entire account structure.
Don’t just stick with one aggregation and assume that that is sufficient to characterize your demand situation fully. Reviewing demand aggregations around different attributes will provide you with greater insight into your business.
Generally, there is a trade-off between effort and accuracy when choosing your attribute level. Top-down analysis is low-effort, but forecast accuracy is mixed. More granular assessment is more accurate, but it takes more effort. Going right down to the item-level is a high effort but also low accuracy in many cases where data are sparse.